2 Aug 2024
Updated on January 31st, 2025
Generative AI vs Predictive AI: Choosing the Right Technology for Your Business
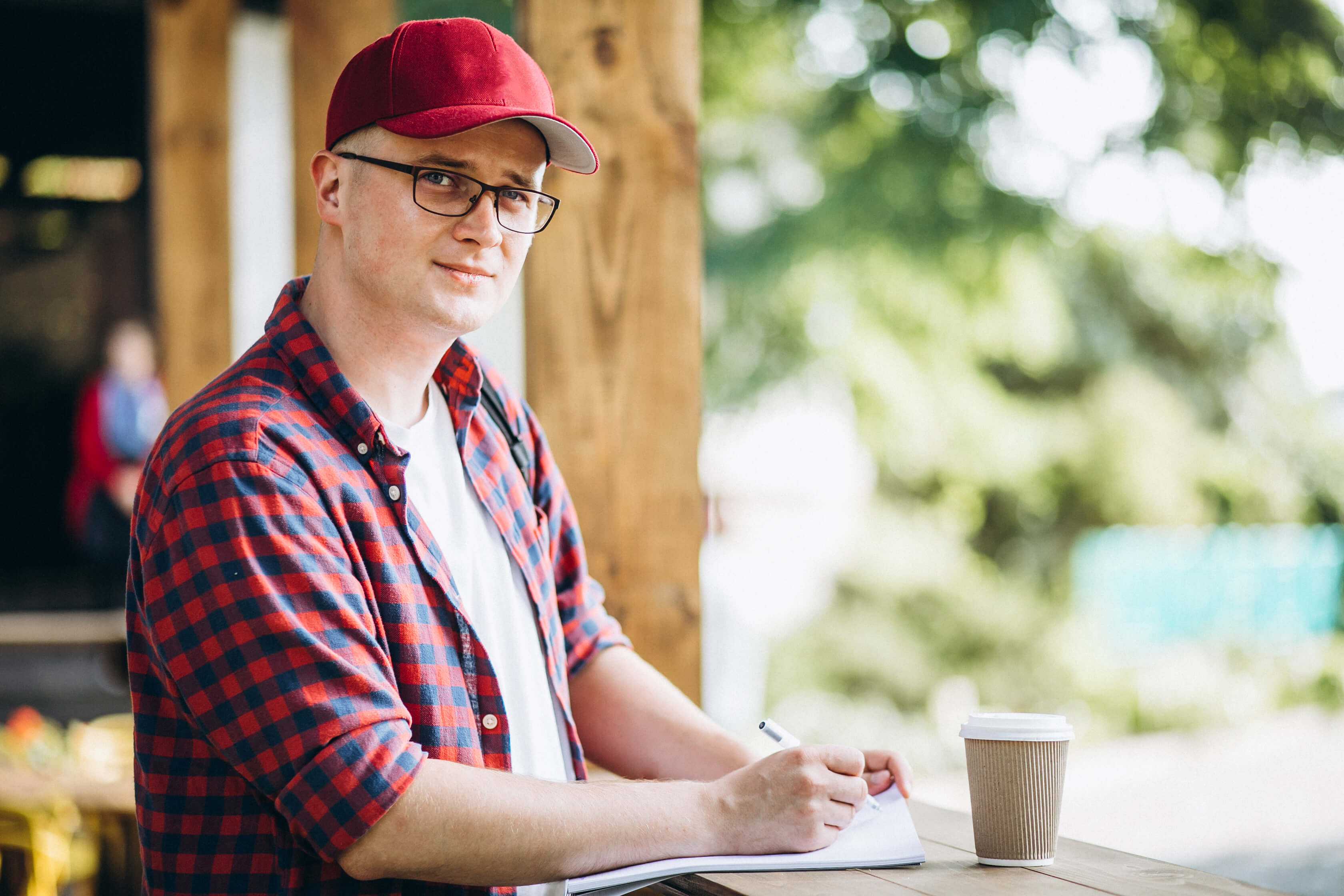
Matthew Connor
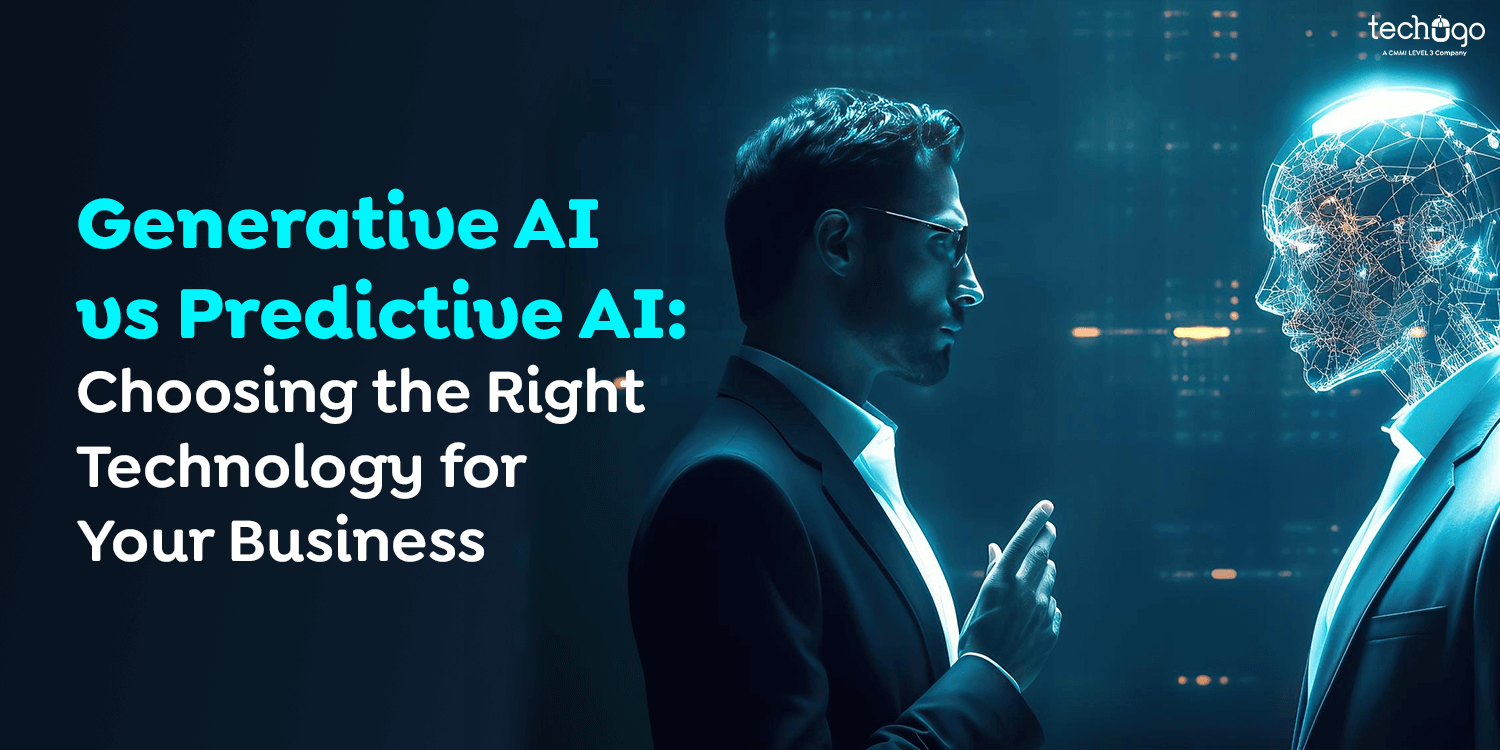
Artificial intelligence, or AI, is an increasingly popular tool for millions of tech and internet users.
Artificial Intelligence (AI) has evolved from a simple concept in science fiction to an active driver of real-world innovation. It is now a significant force in business, industries, and daily life.
In this era of rapid technological advancement, two major branches of AI have come to prominence as game changers: Generative AI and predictive AI. Each is a distinct approach and technology with distinctive capacities and uses.
Generative AI is renowned for its capacity to produce brand-new content that has never been seen before, and Predictive AI excels at forecasting future outcomes based on current data.
This blog will explore the two kinds of AI: generative AI vs predictive AI. Both are useful for work processes and tasks. Understanding the differences between them is essential for knowing when to use each.
Exploring Generative AI
It is an area of artificial intelligence that provides software or programs that create inventive elements through machine learning. Some of the most successful examples of tools based on Generative AI include ChatGPT and Gemini from Google. In the same way, Midjourney is an example of a Generative AI-based tool for creativity that assists users in creating images with a simple call to action.
Furthermore, Generative AI-based software can create images, text, video, code, and recognize voice commands. The input comes in requests that users provide to let the AI recognize what they need from the output. The prompt serves as a means to communicate your needs to the AI, enabling it to benefit from its capabilities and build context from data.
The dynamic AI definition exposes its ingenious nature, driven by cutting-edge techniques, including:
Generative Adversarial Networks (GANs)
GANs comprise two neural networks – a generator and a discriminator. The generator generates new data samples while the discriminator compares the samples against actual data. It then improves over time to produce very real data. The process, similar to a game of cat and mouse, increases the ability of the generator to create realistic data that could fool the discriminator.
Variational Autoencoders (VAEs)
VAEs are another advanced neural network design in Generative AI. They convert input data to a simpler, less latent representation, then reassemble it into data that is similar to the original. This allows VAEs to create fresh, original content using the patterns that they learn through training.
Use of Generative AI
Generative AI is used in the educational setting because staff and faculty can use it to design unique student material to aid learning.
Students can also utilize this technology to assist them with their writing and essays. Generative AI can also assist in making decisions, such as summarizing meetings, producing code, and generating visual artifacts. Although users depend on Generative AI in various ways, you’re probably already familiar with one of the most specific, flexible tools, such as ChatGPT.
ChatGPT is an automated text-generating AI tool developed by OpenAI. It is a type of dynamic AI that numerous users have integrated into their everyday technology usage.
In particular, ChatGPT compiles information from its analysis of previous textual content on websites and other online sources. The AI program uses the information to create unique sentences to talk to you.
Benefits of Generative AI for Businesses
In its essence, the generative AI concept is all about generating new possibilities in digital art and communication. Here are a few advantages to illustrate its significance:
Creativity Amplification
Generative AI increases creativity by inventing innovative ideas, designs, and solutions. It assists artists, designers, and marketers in developing original content, from music and artwork to new product designs that increase creativity and innovation.
Hyper-Personalization
Generative AI enables hyper-personalization by analyzing individual customer data to create tailored experiences. These include customized marketing campaigns, customized product suggestions, and personalized customer service experiences that increase customers’ satisfaction, loyalty, and retention.
Data Synthesis
Generative AI is skilled at creating synthetic data that is very similar to real-world datasets. This is particularly important for creating machine learning models when data is in short supply, expensive, or carries privacy issues.
Adaptive Learning
Generative AI applications can adapt to and even learn from new data inputs, continuously enhancing their efficiency. Their adaptive learning feature ensures that AI models remain current and efficient, even when they encounter changing and new data patterns.
Innovation in Product Development
Generative AI enables new services and products to be created through rapid prototyping and iterating ideas. This accelerates the development timeframe and allows businesses to launch new products more quickly and stay ahead of their competitors.
Time and Cost Savings
Generative AI drastically reduces the time and expense associated with these tasks by automating content production and data generation. Companies can create quality outputs faster and with fewer resources, which increases their efficiency.
Better Productivity
Generative AI improves business workflow eff, efficiency, and effectiveness. It is a perfect way to automate repetitive tasks, allowing employees more time to concentrate on creative and strategic work, which improves overall productivity.
Realistic Simulations
The term “generative” refers to AI, which defines the role of technology in creating realistic scenarios and environments for various purposes. In the aerospace and automotive industries, playing around with various situations and conditions is more convenient without the risk and expense of real trials.
Enhanced Knowledge
Generative AI assists in organizing and uncovering information by drawing insights from huge databases. It is able to detect patterns, correlations, and trends that human analysts could ignore, providing insights into strategic decisions.
Also Read: Explore How AI in Banking Transforms Customer Service and Security
Challenges Related to Generative AI Implementation
In reality, 80% of the generative AI, instead of predictive AI initiatives, fail at the piloting phase. To prevent this, AI engineers have to overcome certain issues, like:
Unpredictability of Outputs
The content generated by artificial intelligence is sometimes unpredictable, and the outcomes could be low-quality or wrong. It is difficult to trust only AI to perform critical tasks without human supervision. To minimize these risks and ensure high output quality standards, it is vital to employ rigorous quality control measures to keep a constant eye on.
Ethical Considerations
Generative AI can produce extremely realistic but possibly inaccurate content, such as deep fakes. These artificially produced videos, images, or audio recordings resemble real people, usually very convincingly.
There are many ethical implicit technologies that could be used to spread false information, commit fraud, or violate privacy. To resolve these ethical issues, it is imperative to create robust detection methods and specific guidelines and rules to regulate the usage of Generative AI.
Computational Resource Requirements
Deep-learning-based, generative AI models require a large amount of computational resources and power. The process of creating models like these can be time-consuming as they require the latest equipment, like GPUs and TPUs, and large quantities of energy.
This high resource demand can challenge small companies or those with budgetary restrictions. Optimizing AI models to increase efficiency and exploring cloud-based solutions can help manage resource demands.
Unlocking Future Trends: The Power of Predictive AI Unveiled
Defining Predictive AI and What it is Used For?
Predictive AI concentrates on forecasting whether trends, patterns, or even the timing of events. Similar to generative AI and predictive AI, predictive AI employs machine learning to improve and adapt, enhancing the customer experience and forecasting the future for businesses.
However, while generative AI uses ML to produce new content, predictive AI employs ML for statistical analysis to predict future events. In contrast to neural networks, predictive AI uses simpler models to gather huge amounts of data, often called “big data,” and make predictions based on the data.
The main purpose of predictive AI is to detect future trends or patterns, which aids in managing inventory, control of the supply chain, campaigns for marketing, and user experience. By studying the previous activities of your employees or clients, predictive AI will assist you in determining what products require replenishment or anticipating user behaviors and help you make health-related choices.
Predictive AI additionally uses embeddings to store data and discover relationships between data sets. Then, it utilizes these relationships to create predictions of future trends. For instance, predictive AI can use embeddings to save purchase history to determine patterns regarding the items customers are likely to purchase soon and when they’ll purchase them. Finding patterns in datasets can help predictive AI assist your business with identifying patterns.
Also Read : How an AI App Development Company Drives Innovation in Mobile Applications
Benefits of Predictive AI for Businesses
Like generative AI, predictive AI offers unique benefits. Some of the benefits that come from prescriptive AI include:
Predicting Human Needs
With its ability to predict the future and future trends, predictive AI can also predict the needs of customers. It can predict your customers’ behavior and purchase habits and guide you on how to advertise new services or products to your existing customers.
In addition, predictive AI can evaluate students’ needs and help determine the ideal time to provide assistance. By offering early interventions to problems with learning, Predictive AI can help create customized studies for students.
More Time to Focus
Predictive AI’s ability to analyze data and anticipate the future makes it useful for managing business processes. Predictive AI can complete quick-term tasks by analyzing data, and your employees will focus their attention on decision-making and imaginative decisions. Automating administrative-centered processes in the workplace with predictive AI can help you devote more time to complex activities that require human intellect.
Data-Driven Decisions
Predictive AI enables businesses to make decisions based on data instead of intuition. By looking at historical data and spotting patterns, companies can help predict future outcomes with greater accuracy and make informed decisions, reducing the chance of costly mistakes.
Cost Optimization
Predictive AI assists businesses in optimizing their resource allocation by predicting trends for the future and identifying issues that could arise prior to their onset. For instance, predictive maintenance can predict the possibility of equipment failure, which permits prompt interventions to avoid expensive downtime and prolong the life of equipment.
Advanced Risk Management
Predictive AI improves the risk management process by identifying potential weaknesses and threats across various domains, such as cybersecurity risks, financial risks, and disruptions to supply chains. This proactive approach permits organizations to adopt proactive measures to reduce risks efficiently.
Competitive Edge
Predictive AI can give businesses an advantage in competition by allowing them to anticipate market trends and consumer behavior. With this insight, businesses can innovate, rapidly adapt to changing conditions, and maintain an edge over the competition in a rapidly changing market.
Innovation in Product Development
Predictive AI helps in product development by studying market trends and customer feedback to forecast customers’ future requirements. This allows companies to develop items and products that match their customers’ changing needs to ensure they are relevant and innovative.
Operational Efficiency
Predictive AI automatizes complex data analysis, dramatically cutting down on the time and effort needed to perform manual analysis. Automation improves efficiency, which improves productivity and lets employees focus on the most valuable tasks that require human knowledge.
Personalized Customer Experiences
Predictive AI analyzes customers’ habits and preferences to provide individual customer experiences. Businesses can customize their marketing strategies, products, recommendations for products, and interactions with customers to meet specific needs. This increases customer satisfaction and loyalty.
Strategic Resource Allocation
With the help of predictive data, companies can better use their resources. For example, predictive demand forecasting can help retailers improve their inventory levels to ensure they can satisfy customer demands without under- or overstocking.
Enhanced Marketing Precision
Predictive AI improves marketing strategies by studying consumer data and predicting consumers’ future behavior. This makes creating precisely targeted campaigns that connect with the public, boost engagement, and make each dollar count easier.
Also Read : AI in Mental Health: Discover the Challenges and Opportunities
Challenges in Implementing Predictive AI
Predictive AI can provide significant advantages; however, its effective implementation can be challenging. Here’s a more detailed analysis of what companies could confront:
Dependence on Data
Predictive AI heavily depends on the quality and accuracy of past data. Predictions will not be reliable when incorrect data has missing parts or bias. The reliability of AI performance is dependent on continuous data cleansing, validation, and updates, which can be time-consuming and difficult.
Problems which Bias as well as Fairness causes
Predictive AI models may take on biases in the data they are trained on, which can result in inaccurate or biased outcomes. If, for instance, the historical data has social biases, the AI might reflect these biases when making predictions. To tackle this issue, AI engineers must carefully examine data, conduct frequent audits, and use fairness-aware methods to ensure the AI is ethical and fair.
Scalability and Adaptability
Predictive AI models may struggle with adaptability and scalability. To ensure accuracy, they should be kept up-to-date and trained regularly in response to the changing business environment and data patterns.
In addition, scaling predictive AI applications to deal with greater amounts of data and more complicated scenarios is challenging. This requires a robust infrastructure, large computational resources, and a strategic approach to managing models and their deployment.
Generative AI vs Predictive AI: The Major Differences
Generative AI vs Predictive AI differs in functions, though their applications sometimes overlap. Are you eager to understand what these technologies can do for your business? Below, we’ve compared capabilities, AI app, and the difference between generative AI and Predictive AI.
-
Purpose
Generative AI
The principal goal of Generative AI is to produce new information or content that was not present before. This means creating completely new outputs such as texts, images, music, or even designs created from scratch according to the patterns and examples it learned from. This results in Generative AI being a powerful tool for innovative industries and projects.
Predictive AI
However, Predictive AI studies the data available to anticipate future outcomes. Its primary goal is to predict patterns, behavior, or events by identifying patterns and forming informed predictions. This AI is used extensively in healthcare, finance marketing, and supply chain management.
-
Core Functionality
Generative AI
The primary function, which is the core function of Generative AI, revolves around using sophisticated algorithms to comprehend and reproduce patterns within the data it has trained, allowing it to create new and distinctive content. Models such as Generative Adversarial Networks (GANs) and Variational Autoencoders (VAEs) are common examples. In these models, GANs utilize a two-model model (generator and discriminator) to produce authentic data.
Predictive AI
Predictive AI leverages machine learning and statistical model algorithms to analyze the past and make accurate forecasts of the future. Methods like the regression model, decision trees, and neural networks are typically employed to create these models. They can provide information and forecasts.
-
Output
Generative AI
The results generated by Generative AI are often creative and varied, and they include writing articles, creating new artworks, synthesizing voice recordings, and many more. One of the unique aspects of generative artificial intelligence is that the generated content is usually unique, which means it has not been created before. This is why it’s important in fields that demand imagination and originality.
Predictive AI
Predictive AI produces outputs from probabilities, insights, or predictions. These outputs are based on analysis of historical data and provide useful foresight. The typical outputs are sales forecasts, risk assessments, customer behavior forecasts, and more to aid in making strategic decisions.
-
Data Requirement
Generative AI
Generative AI requires large and diverse data sets to create realistic and high-quality media. The more diverse and extensive the data is, the more efficiently the AI can learn and replicate intricate patterns. This large amount of data is required to ensure that the outputs generated are as accurate and authentic as possible.
Predictive AI
Predictive AI relies heavily on historical data, focusing on its quality and relevance rather than just volume, as predictive accuracy depends on the algorithm being fed data closely matched to the conditions it aims to predict.Therefore, tidy, reliable, well-organized, and unbiased data sets are essential for successful predictive modeling.
-
Use Cases
Generative AI
Generative AI can be found in creative industries, such as content, entertainment, and design. For instance, it can make realistic human faces, write coherent narratives, design new products, or even write music. These applications demonstrate its ability to create innovative and interesting content.
Predictive AI
Predictive AI is used extensively in areas that require forecasting and trend analysis. It predicts financial markets’ stock prices and healthcare, diagnoses health issues, analyzes customer behavior in marketing, and maximizes supply chain processes. The accuracy of its forecasts makes it a valuable tool for strategic planning and decision-making.
-
Model Training
Generative AI
The training process for Generative AI models typically involves complex processes, including the adversarial learning process in GANs, which involves two models (a generator and the discriminator) being simultaneously trained. Generators create data, while the discriminator analyzes its authenticity, resulting in an improvement in the model over time. This process is computationally demanding but produces top-quality outputs.
Predictive AI
Learning Predictive AI models typically involves mixing historical data into a single model to minimize the possibility of error in prediction and increase accuracy. The training process focuses on adjusting the parameters of the model to increase its ability to produce accurate forecasts on the latest data. Techniques like cross-validation and hyperparameter tuning are frequently used to improve predictive models.
-
Feedback Mechanism
Generative AI
Generative AI utilizes a unique feedback mechanism known as adversarial-based training. This method constantly enhances generator models using input received from the discriminator, which measures the quality of the output. This feedback loop is dynamic and aids in improving the quality of output over time, making generated data more accurate and sophisticated.
Predictive AI
Predictive AI is based on a feedback mechanism in which actual outcomes are compared with predictions. The model is continuously updated and refined based on the feedback received, allowing it to make mistakes and improve its future predictions. This ensures that the model’s predictive accuracy grows more precise and reliable as time passes, increasing its usefulness in real-world applications.
-
Evaluation Metrics
Generative AI
The assessment of Generative AI models is based on the authenticity, novelty, and quality of the generated content. Common metrics are the Inception Score (IS), which evaluates the variety and quality of the generated images, and the Frechet Inception Distance (FID), which measures the image’s distribution to real-world images. In addition, the human eye is commonly used to judge how subjectively good generated content is.
Predictive AI
Predictive AI models are analyzed using various statistical metrics to assess their performance and accuracy. Common measures include precision, accuracy recall, F1 score, the Mean Absolute Error (MAE), and the Root Mean Squared Error (RMSE). These metrics offer a quantifiable indicator of how well the predictive model is performing in identifying areas of improvement.
-
Computational Complexity
Generative AI
Generative AI models are typically more intensive in computational terms because of the difficulty required to create real-looking and high-quality outputs. Models for training like GANs are particularly resource-intensive and require a significant amount of computation power and a long time. The computational complexity is in exchange for the possibility of creating new and varied content.
Predictive AI
While predictive AI models may be extremely computationally intensive, they are typically less demanding than generative models. The level of complexity depends on the particular algorithm used and the data being used. Strategies like decision trees and simple regression models can use less computational power, while deep learning models may be more complex.
-
Ethical Considerations
Generative AI
Generative AI poses ethical issues primarily concerned with the authenticity and possible misuse of created content. Problems like deep fakes, in which realistic fake videos or images are made, pose significant dangers. The use of ethical methods and the prevention of the misuse of Generative AI technologies are crucial for maintaining trust and integrity.
Predictive AI
Predictive AI has ethical issues that concern fairness and bias in its predictions. It is important to ensure that predictive models don’t reinforce existing biases and that the predictions they make are accurate for different groups of people. Transparency and accountability when predictive models are designed and used are essential to address ethical concerns.
-
Technological Challenges
Generative AI
One of the major issues facing Generative AI is creating high-quality content comparable to actual data. Preventing mode collapse, in which the model can only produce very few variations, and managing the massive computational load needed to train are also problems. Continuous improvement and innovation are needed to conquer these technological obstacles.
Predictive AI
Predictive AI faces challenges such as dealing with large and complex data, improving prediction accuracy, and tackling issues such as overfitting, in which the model can perform well on training data but fails to perform well on new data. The ability to interpret predictive models, which means that users comprehend the process of making predictions, is a major challenge.
-
Examples
Generative AI
A few examples that demonstrate Generative AI include OpenAI’s GPT-4, which generates human-like text; DALL, which creates images using textual descriptions; and DeepArt, which transforms images into different artistic styles. These examples demonstrate the flexibility and creative possibilities of Generative AI in creating interesting and unique content.
Predictive AI
A few examples of Predictive AI include models for forecasting weather that can predict forecasts for weather patterns, economic market forecasting systems that forecast prices for stocks, and recommendation algorithms utilized by companies like Netflix or Amazon to recommend the best content for users. These apps demonstrate the utility of Predictive AI in providing accurate and actionable information.
The Key Takeaway
Let’s look at the previous points – Generative AI vs Predictive AI. Predictive AI lets you know what’s likely to happen Generative AI enables you to think ahead and respond. You’ll get the most effective technology and foresight that work together.
AI development is heading towards more advanced models and better capabilities. Understanding the differences between predictive and generative AI is vital for an app development company like Techugo to utilize these tools effectively in various industries.
The ongoing advancement of artificial intelligence provides many opportunities for professionals seeking to specialize in this area. The future holds enormous potential for technological advancements, both predictive and generative AI, which indicates exciting possibilities for growth and innovation in many different industries across the globe.
Get in touch with us today to begin a journey towards digital transformation and excellence together.
Get In touch
We are excited to here from you and let’s start something special Together. Call Us for any inquiry.
Write us
sales@techugo.caJust a call away
About you
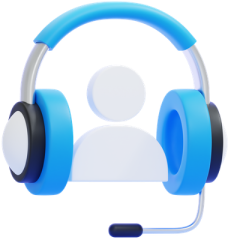